
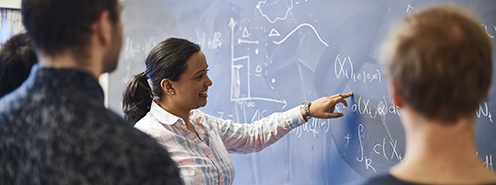
Statistics and Probability Theory
The research in the section covers topics ranging from probability theory and mathematical statistics to applied statistics.
Main themes in our research include
- Markov processes,
- biostatistics, biomathematics,
- inference for statistical models based on stochastic processes,
- general principles for statistical inference,
- graphical models and their applications (see CoCaLa homepage),
- asymptotic statistical theory,
- functional data analysis, and
- applications of statistical methodology and stochastic processes in bioinformatics, neuroscience, systems biology, reaction networks (see MBIO homepage), physiology, and earth science.
In particular, the section works with statistical inference from discrete time sampling of continuous time processes, such as models given by stochastic differential equations and jump processes, or from first-passage-time data from these models. Also, statistical learning and inference for point processes are studied, as well as Monte Carlo simulation, hidden Markov models. Especially gene expression data, DNA data and stochastic neuronal models are studied.
Mathematical Biology (MBIO)
Copenhagen Causality Lab (CoCaLa)
Susanne Ditlevsen
Elisenda Feliu
Ernst Hansen
Niels Richard Hansen
Steffen Lauritzen
Bo Markussen
Jonas Peters
Helle Sørensen
Michael Sørensen
Anders Tolver
Carsten Wiuf
Contact information - all section members
The section hosts Data Science Laboratory together with the Department for Computer Science. The lab develops and applies statistical methods in close collaboration with researchers from other departments at the faculty.
Prospective students are encouraged to visit the study website
Master of Science (MSc) in Statistics
Current students can visit MCs in Statistics on the student intranet.
Contact
Professor
Niels Richard Hansen
Head of Section
Office 04.3.18
E-mail: niels.r.hansen@math.ku.dk
Phone: +45 35 32 07 83
Mobile: +45 28 59 07 65